Nearly twenty years ago the FDA approved the ABL inhibitor imatinib to treat leukaemia’s with BCR-ABL fusion. Proof that the specific genomic alterations of a cancer can be targeted therapeutically fundamentally changed cancer research and sparked an explosion of research into precision therapies. Unfortunately, a revolution in cancer science has not yet led to a revolution in cancer treatment. Most patients do not have an approved indication for targeted therapies, and many of those that do have variable and temporary responses to treatment.
The goal of precision oncology is to assign every patient a therapy regimen that will selectively kill neoplasm while sparing healthy tissue. This daunting undertaking will require a radically expanded understanding of cancer biology. In particular, we need to identify many more cancer vulnerabilities and selective dependencies that can be exploited therapeutically. The Cancer Dependency Map (DepMap) project is a strategic collaboration between the
Broad and
Sanger Institutes aimed at accelerating these discoveries. One of the cornerstones of this effort are cancer genome-wide CRISPR knockout viability screens in hundreds of cancer cell lines to identify selective genetic dependencies. Known as
Project Achilles at the Broad and
Project Score at the Sanger, these efforts were initially conceived and carried out independently, using different methodologies, with some overlapping and some mutually exclusive cell lines. This provided a unique opportunity to evaluate the reproducibility of CRISPR viability screens at scale, which is the subject of our
first Broad/Sanger DepMap joint paper. Finding that the screens are highly reproducible motivated us to create a unified dataset, which is the subject of our
second joint paper.
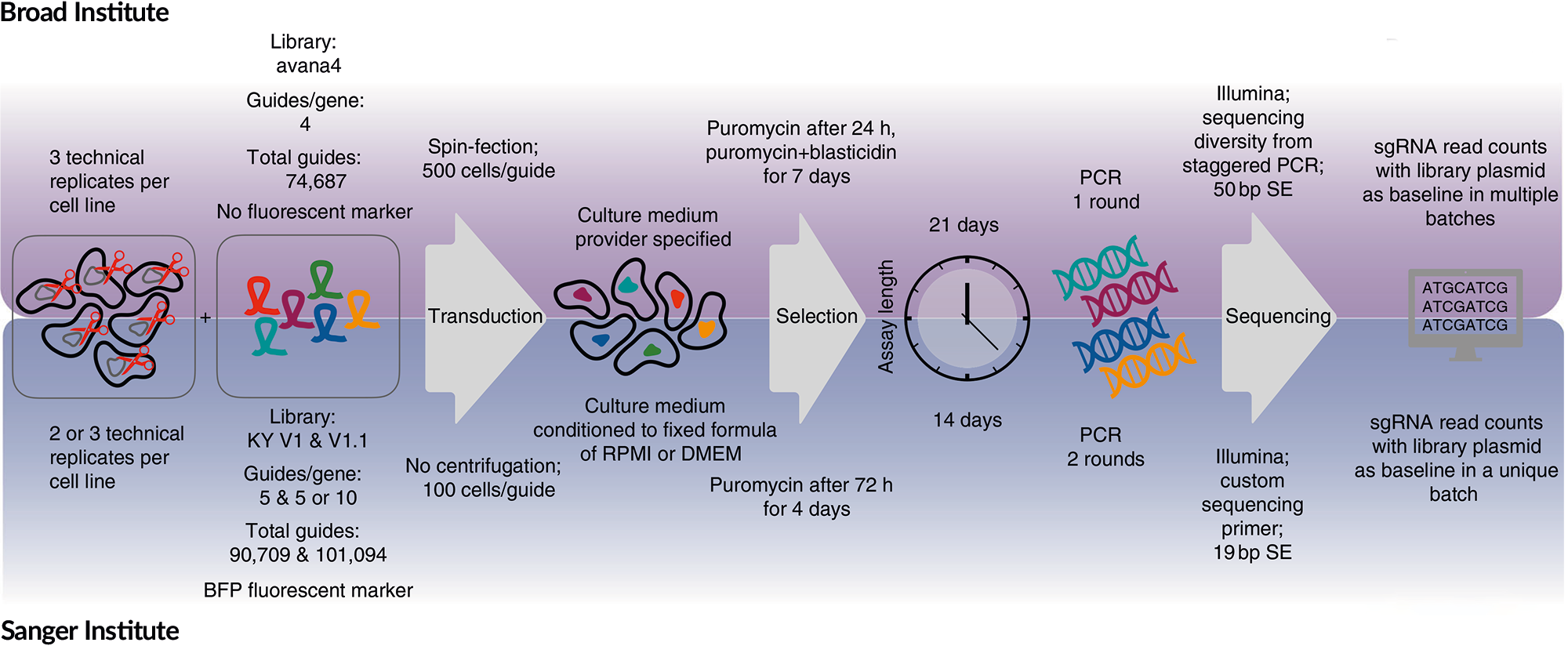
The experimental pipelines underlying the Achilles and Score projects were different at nearly every step. Strategies for cell infection, selection, passaging, media choice, library design, screen duration, PCR amplification, and sequencing methods all deviate between the two projects. Additionally, each institute had its own methods for creating gene fitness scores and correcting for copy number bias:
CERES at the Broad, and
CRISPRCleanR at the Sanger. These differences create challenges from a data perspective but also have some advantages. If we find reasonable agreement between the datasets, when all these parameters vary, we can offer fairly robust guarantees about the reproducibility of CRISPR screens. Benchmarking is particularly important given
concerns about RNAi screens and the debate about the reproducibility of cell line drug screens.
We analyzed the agreement between Achilles and Score dependency datasets along multiple axes and found good concordance. The Pearson correlation of all gene dependency scores was 0.658, rising to 0.765 with ComBat batch correction. Both studies identified similar sets of common essential genes (1,031 common essentials were identified by both institutes out of 1,688 identified by either). Importantly for precision medicine, both studies not only identified consistent sets of biomarkers for selective dependencies, but also agreed on their predictive power. The data we used for this study, including intermediate results, can be found on
FigShare, and the code used to conduct analyses and create figures on
github.
The agreement of Broad and Sanger gene dependency scores from genome-wide CRISPR screens
In red are the essential genes identified by Hart et al. Right: Pearson correlation of a gene’s dependency score (upper label) with a second gene’s expression (lower label) found from each institute’s dataset, for genes with strongly selective dependency profiles across cell lines in at least one institute. Gene expressions with strong correlation to the dependency of interest are potential biomarkers.
In our comparative analysis, we found good concordance but also clear batch effects between the Achilles and Score datasets. In order to integrate them into a large, unified dataset of cancer dependencies these effects had to be removed. In our
second paper, we studied the effects of different processing steps and different batch correction methods on the data.
Broad/Sanger cancer dependency data integration strategy
a-b. Gene dependency datasets (raw count data of single-guide RNAs) are pre-processed with three different methods, accounting for gene-independent responses to CRISPR-cas9 targeting (arising from copy number amplifications) and heterogeneous sgRNA efficiency, providing gene-level corrected depletion fold changes. Then, four different batch-correction pipelines are applied to the gene level fold changes across the two institute datasets for each of the pre-processing methods. c. Twelve different integrated datasets resulting from applying three different pre-processing methods and four different batch-correction pipelines are benchmarked. d. Three different integrated datasets obtained by applying three pre-processing methods and the best batch-correction pipeline are benchmarked. e. Advantages provided by the final integrated datasets and conservation of analytical outcomes from the individual ones are investigated.
Although standard batch correction methods can be used to align the datasets, it is important that these preserve the heterogeneity of gene fitness effects in different cell lines which are attributable to biological factors. Using multiple metrics, we validated the ability to retain the heterogeneity in the data and assessed the extent of biomarkers recovered pre/post batch correction.
Alternative methods to correct for CRISPR-Cas9 specific biases, such those arising from targeting copy number altered genes and from variable guide efficiencies, also result in differences between datasets. To aid interpretation of integrated CRISPR-Cas9 screens we also examine differences resulting from using various pre-processing methods.
The
integrated datasets span over 900 cell lines, representing the largest integrative resource of genetic dependencies in cancer. Using the integrated datasets, we identify 2,103 common essential genes with associated confidence levels. Further, we found biomarkers of dependency only identifiable in the integrated data sets and not in the individual datasets.
These collaborative studies are helping to establish standards and benchmarking strategies for combining and integrating cancer dependencies datasets. This is laying the foundation for efforts to map dependencies across a wide range of patient tumours representing the genetic, ethnic and clinical diversity of human cancer.